Agentic systems handbook: Benefits and use cases
Agentic systems are artificial intelligence (AI) networks capable of independent action toward defined goals.
What you need to know:
- Agentic AI systems operate and adapt on their own, mimicking human-like decision-making to achieve specific goals without constant human oversight.
- Agentic systems are reshaping business operations by automating complex workflows. They deliver high efficiency and 24/7 availability at scale.
- Successful adoption requires establishing clear governance and ethical guidelines to address concerns about AI bias, privacy, and transparency.
- Automation Anywhere integrates AI agents with strong automation capabilities. The platform provides tools for monitoring and refining AI behavior for security and responsible use.
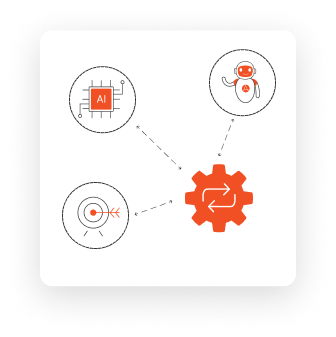
What are agentic systems?
Characterized by autonomy, decision-making capabilities, and adaptability, agentic systems are AI networks that can reason through complex scenarios and adjust what they do to achieve specific objectives.
This goal-oriented behavior is a key feature of agentic systems. Designed to pursue outcomes without constant human intervention, they work dynamically—taking in data from various sources, evaluating potential actions, and executing strategies—mimicking human-like decision-making processes to determine next steps.
While non-agentic AI operates reactively within a defined framework—such as identifying objects in images, recommending products based on browsing history, or responding to voice prompts with information—agentic systems make decisions and take action without explicit direction.
In this way, agentic systems are more like AI colleagues than assistants; they’re partners in achieving complex tasks and goals. Not just following commands, they’re capable of setting course and adapting to new information on their own, using memory frameworks to retain and apply knowledge from past interactions.
A core component of an agentic system is the AI agent—an autonomous entity assigned to perform specific tasks.
Tour the secure Agentic Process Automation System
Benefits and implications.
Implementing agentic AI systems offers transformative benefits in enterprise environments. By combining autonomy, adaptability, and real-time decision-making, agentic systems enable the automation of complex workflows and multi-step processes with minimal oversight, delivering unprecedented efficiency and scalability.
Increased operational efficiency and reduced human error
Agentic systems are designed to automate complex workflows and repetitive tasks, leading to improved operational efficiency and minimizing human error, which is often a byproduct of manual processes.
24/7 availability and scalability of AI agents
One of the standout features of automated systems is their ability to operate around the clock without the constraints of fatigue or downtime. This constant availability allows organizations to maintain continuous service and support.
And with agentic systems, AI agents can seamlessly scale to handle increasing volumes of tasks or interactions, providing elastic capacity, which is particularly advantageous during peak business periods.
Ability to process and act on vast amounts of data in real time
Thanks to their underlying large language models (LLMs), agentic systems excel in gathering and analyzing large volumes of data quickly. This capability helps make informed decisions based on real-time insights.
Potential for innovative solutions through AI-driven insights
The integration of agentic systems can lead to the development of innovative solutions driven by analyzing patterns and trends to identify opportunities for new products or services.
Impact on workforce dynamics
The benefits of implementing agentic systems are substantial, but making the transition to AI-powered operations requires embracing these systems thoughtfully.
While the implementation of agentic systems presents numerous opportunities—from assisting in patient triage by analyzing electronic health records to prioritize care, to identifying and neutralizing cybersecurity threats in real time—it also poses challenges ranging from concerns about job displacement to data privacy and the ethical use of AI.
Addressing these known and emergent issues proactively and instilling a culture of transparency and inclusivity will be foundational to successfully achieving AI-driven operations.
Human-AI collaboration
As agentic systems become more prevalent, the dynamics of the workforce are likely to shift towards models of human-AI collaboration. Rather than viewing AI as a replacement for human workers, organizations can harness the strengths of both to achieve better outcomes.
Agentic AI has the potential to augment human capabilities by taking over mundane tasks, enabling employees to engage in more strategic and impactful work. This division of labor boosts both job satisfaction and overall productivity.
For example, in customer service, AI agents can free human agents to address complex issues that require empathy and nuance. Agentic AI can also expand the possibilities of human projects, augmenting biomedical research with out-of-the-box thinking and unconventional strategies.
New roles and skills
The proliferation of agentic AI will inevitably lead to the emergence of new roles and skill sets. Roles like AI orchestration specialists, data ethics officers, and automation strategists are set to become integral to organizations adopting agentic technologies in order to align AI models with specific goals, maintain ethical standards, and integrate AI with existing systems.
And in general, employees may need training to develop competencies in managing and collaborating with agentic systems, as well as in data analysis and interpretation.
Applications of agentic systems.
Agentic systems are increasingly being adopted across industries, demonstrating versatility and effectiveness in optimizing complex workflows and enhancing operational efficiency. Real-world applications of agentic systems span a diverse range of use cases, showing how they address specific challenges in different sectors.
Financial services
- Automated trading strategies: Agentic systems can analyze market trends and execute trades at high speeds, optimizing investment portfolios. For example, hedge funds use algorithmic trading strategies that leverage AI to make split-second decisions based on real-time market data, resulting in significant profit margins.
- Fraud detection and containment: AI agents analyze transaction patterns to identify anomalies that may indicate fraudulent activities, such as unusual spending behaviors, initiating rapid intervention procedures.
- Personalized financial advice: Agentic systems can provide tailored financial recommendations based on individual client profiles, investment goals, and risk tolerance. So-called robo-advisors can set up and rebalance user portfolios based on personalized investment strategies without the need for human financial advisors.
Healthcare
- Patient triage: AI-driven systems can assess patient symptoms and prioritize care based on urgency. For example, agentic systems integrated into healthcare platforms can conduct initial evaluations via chatbots, directing patients to appropriate care facilities.
- Treatment planning: Agentic systems analyze patient data and medical histories to suggest optimal treatment plans. IBM Watson Health is an example, utilizing agentic AI systems to assist oncologists in developing personalized cancer treatment strategies.
- Drug discovery: Agentic systems accelerate the drug discovery process by analyzing biological data and predicting which compounds will be effective. Companies like Merck significantly accelerated compliance processes, reducing barriers to bringing new drugs to market while saving 150,000 hours annually.
Manufacturing
- Supply chain optimization: As part of an agentic system, AI agents can forecast demand and adjust inventory levels, minimizing excess stock and reducing costs. For instance, companies like Unilever and Siemens use AI to predict supply chain disruptions and optimize logistics, leading to more efficient operations.
- Predictive maintenance: By monitoring equipment performance and analyzing historical data, agentic systems can predict when machines are likely to fail, enabling timely maintenance.
- Quality control: Agentic AI systems can analyze production processes in real time to detect defects and ensure product quality. For example, real-time data from computer vision systems to inspect products on assembly lines can feed agentic systems to initiate immediate corrective actions and improve overall quality.
Customer service
- Advanced chatbots: AI-agent-driven chatbots can independently handle customer inquiries and provide instant responses to improve service efficiency, and conversational automation offers instant access to action AI agents.
- Personalized support: Agentic systems analyze customer data to offer tailored support solutions. For example TaskUs realized efficiency and productivity gains with AI-powered assistance for frontline agents, improving AHT 105% over target.
- Issue resolution: Agentic automation with AI agents can categorize and prioritize support requests, ensuring that urgent issues are addressed right away.
Marketing
- Globalization: AI agents automate and streamline globalization operations, helping teams cut localization costs by over 50%, deliver faster content translations, reduce manual workloads, and provide consistent, high-quality regional experiences.
- Campaign optimization: An agentic AI system can analyze buyer behavior and engagement metrics to refine marketing campaigns in real time. They can generate personalized email campaigns or optimize ad placements in real time, ensuring marketing efforts align with goals and enhance engagement.
- Content creation: AI-agent-powered tools can generate and deploy marketing content based on analyzing market data to identify emerging trends and consumer sentiment.
Optimizing complex workflows
The benefits associated with implementing agentic systems primarily stem from their ability to optimize complex workflows through advanced automation, real-time data processing, and intelligent decision-making.
By leveraging machine learning algorithms and AI-driven insights, agentic systems can analyze data, identify patterns, and make informed decisions, which allows them to automate repetitive tasks, handle exceptions without human intervention, and coordinate multiple systems or functions seamlessly.
As a result, organizations experience increased operational efficiency, reduced human error, and enhanced responsiveness even amid changing conditions, ultimately driving significant cost savings and improved productivity.
Automating decision-making processes
In scenarios with multiple variables, agentic systems can analyze data and make informed decisions autonomously. For instance, in supply chain management, AI can dynamically adjust orders based on real-time inventory levels and sales forecasts, enhancing responsiveness.
Handling exceptions and edge cases
Agentic systems can manage unexpected situations without human intervention. For example, in finance, AI algorithms can identify and resolve discrepancies in transaction data, ensuring accuracy and compliance.
Coordinating multiple systems
Agentic systems facilitate the seamless integration of various functions and tools to complete end-to-end processes. For instance, in manufacturing, AI can synchronize production schedules with supply chain logistics, optimizing overall efficiency and reducing costs.
Automating sophisticated knowledge work
The role of agentic systems in automating knowledge work is poised to expand dramatically, building on existing applications across business, research, and scientific domains.
Applying advanced algorithms and natural language processing enables agentic systems to both streamline complex tasks and improve decision-making at the same time, empowering professionals by reducing time spent on routine analyses and allowing focus on more strategic initiatives.
The ability of these systems to process vast amounts of information and derive actionable insights is already reshaping industries such as law, healthcare, and technology.
- For example, agentic systems are automating legal research and contract analysis. AI systems analyze legal documents and case law, accelerating the research process.
- Across research fields, agentic systems support literature review and hypothesis generation. Agentic systems can sift through the immense body of scientific publications to identify relevant studies, discover connections between them, and generate hypotheses for researchers.
- For strategic business planning and forecasting in any industry, agentic systems can analyze market trends and internal data to generate forecasts and strategic recommendations.
- Applying agentic systems within software development and debugging assists developers by automatically finding bugs and suggesting code improvements, driving development quality and speed—and turbocharging developer productivity.
The architecture of agentic systems.
Agentic systems are built on interconnected components that enable them to operate autonomously, adapt to environments, and achieve goals. At a foundational level, they rely on prompts, memory, and tools, with each playing a core functional role.
Each component in its operational framework is integral to the system's functionality, allowing it to process information, execute tasks, and dynamically learn from experiences. Understanding these elements helps build a picture of how agentic systems execute complex workflows and provide value.
Core components of agentic systems
Prompts
Prompts are the initial inputs that guide the system’s behavior, ranging from simple instructions to detailed directives. They shape the system’s reasoning and actions. For example, a prompt in healthcare might instruct the system to prioritize patient triage based on real-time data.
Memory
Memory enables systems to retain and use information from past interactions. This allows continuous learning, adapting, and refining outputs over time. In supply chain optimization, memory helps recognize patterns in demand fluctuations, leading to better forecasting and resource allocation.
Tools
Tools extend capabilities by integrating external resources like APIs, datasets, or specialized algorithms. These tools allow agentic systems to perform multi-step operations, from data analysis to automation. In cybersecurity, tools might include threat detection algorithms working with the system’s reasoning engine to identify risks in real time.
Types of AI agents in agentic systems
Agentic systems include networks of different types of AI agents, each designed for specific tasks. At a high level, AI agents fall into three categories:
- 1. Reactive agents focus on immediate responses, ideal for real-time applications like chatbots.
- 2. Deliberative agents emphasize long-term planning, suitable for complex decision-making like financial modeling.
- 3. Hybrid agents combine both approaches, offering adaptability and precision.
Operational steps in agentic systems
Agentic systems operate through a structured series of steps, iterating as needed based on feedback or changes to the environment.
- Step 1: Perception → Gathering inputs from data sources to understand the environment.
- Step 2: Reasoning → Processing inputs using advanced algorithms to draw conclusions or identify solutions.
- Step 3: Planning → Formulating strategies to achieve goals, breaking down workflows into manageable steps.
- Step 4: Execution → Carrying out planned actions, using tools and memory to deliver precise outputs.
- Step 5: Learning → Evaluating performance and incorporating feedback to improve future operations and enhance adaptability.
Step 1: Perception
The first step is perception, which involves gathering inputs from data sources to develop an understanding of the environment. This can include real-time data from sensors, user interactions, databases, and external APIs.
For example, in a financial trading system, perception might involve collecting market data, news articles, and social media sentiment to assess market conditions. The effectiveness of this step directly impacts the system's ability to respond accurately to changes in its environment, as it lays the groundwork for informed decision-making.
Step 2: Reasoning
Once the agentic system has gathered relevant inputs, it moves to reasoning. In this phase, advanced algorithms—such as machine learning models and statistical analyses—process the collected data to draw conclusions or identify potential solutions.
In healthcare, an AI system may analyze patient symptoms and historical data to diagnose a condition. The reasoning step synthesizes relevant information and provides accurate insights to guide subsequent actions.
Step 3: Planning
With insights derived from reasoning, the agentic system enters the planning phase. Here, it formulates strategies to achieve specific goals by breaking down workflows into manageable steps. This may involve creating a sequence of actions or determining resource allocation.
For example, in supply chain management, an agentic system might develop a plan to optimize inventory levels based on forecasted demand and supplier lead times. Effective planning is what enables the system to execute tasks efficiently and align its actions with overarching objectives.
Step 4: Execution
The execution step involves carrying out the planned actions using the tools and memory available to the agentic system. This could include interfacing with other software applications, sending commands to machinery, or delivering outputs to users.
In customer service, a chatbot may execute a plan by retrieving relevant information from a database and providing personalized responses to user inquiries. The execution phase is what translates plans into tangible results, and its success hinges on the accuracy and reliability of the preceding steps.
Step 5: Learning
The final step in the operational cycle of agentic systems is learning. This involves evaluating performance and incorporating feedback to improve future operations. Analyzing outcomes and identifying areas to improve fuels the system’s ability to adapt processes so that it remains effective and relevant in dynamic environments.
For example, an AI-powered marketing tool might assess the effectiveness of a campaign by analyzing engagement metrics and adjusting strategies accordingly.
Implementing agentic systems.
Adopting agentic systems requires a thoughtful approach that considers organizational readiness, data infrastructure, cross-functional collaboration, and strategic alignment with broader digital initiatives.
Assessing organizational readiness and identifying suitable use cases
Getting started with agentic systems involves evaluating the current technology landscape, organizational culture, and available resources.
First, evaluate organizational readiness against technical requirements, including automation and AI capabilities and data infrastructure.
The importance of data infrastructure and quality to agentic systems can’t be overstated. A robust data infrastructure is critical; ensuring high-quality data and integration with real-time sources is necessary for agentic systems to operate at speed and with accuracy.
- Data collection and storage: Establishing reliable mechanisms for collecting and storing data is essential. This may involve integrating existing databases, implementing data lakes, or utilizing cloud storage solutions to facilitate access to relevant information. Optimize data pipelines for both structured and unstructured inputs.
- Data quality management: Ensuring the accuracy, consistency, and completeness of data is paramount. Organizations should implement data governance practices to maintain high data quality, including regular audits, validation processes, and data cleansing techniques. High-quality data enables agentic systems to make informed decisions and deliver reliable outcomes.
- Data security and privacy: Organizations must also address data security and privacy concerns, especially when dealing with sensitive information. Implementing robust security measures and compliance protocols helps protect data integrity and builds trust.
Second, from a strategic standpoint, ascertain whether the goals and objectives for agentic systems are clearly defined.
Whether optimizing operations or automating workflows, clear objectives guide both design and deployment and establish the true north for aligning agentic systems as well as evaluating outcomes.
Key steps to initiating the implementation of agentic systems include:
- Conducting a readiness assessment: Organizations should assess existing capabilities, including technological infrastructure, workforce skills, and leadership support. This evaluation helps identify gaps and areas for improvement that may affect the successful implementation of agentic systems.
- Identifying use cases: Organizations should explore potential use cases where agentic systems can add significant value. This involves analyzing business processes to identify repetitive tasks, complex workflows, or areas that require enhanced decision-making. Engaging stakeholders from diverse departments can provide deeper insight into specific operational challenges and opportunities for AI integration.
- Prioritizing use cases: After identifying potential use cases, organizations should prioritize based on factors such as feasibility, impact, and alignment with strategic goals. Focusing on high-impact areas with achievable outcomes can help build momentum for further AI adoption.
Leadership and cross-functional collaboration
Agentic systems operate at the intersection of technology, business strategy, and human oversight. That means successful implementation requires collaboration across departments like IT, operations, automation leads and, when needed for specialized development and model fine-tuning, data scientists.
- IT teams play a central role in setting up and managing necessary infrastructure, integrating AI technologies, and ensuring system security.
- Strategically, engaging business units ensures that agentic solutions align with operational needs and objectives.
- Operations teams must adapt workflows to incorporate the system’s outputs and reorganize work for seamless human-machine collaboration.
And at the strategic level, leadership involvement can make or break the success of AI initiatives. By supporting initiatives that prioritize adaptability and innovation, executives can create a culture that embraces agentic AI. This often involves reskilling employees to work alongside these systems.
Importance of a comprehensive AI strategy
Agentic systems should be viewed as part of a larger digital strategy driving overall organizational effectiveness. By aligning AI initiatives with digital transformation goals, organizations can ensure that agentic systems contribute to improved processes and outcomes.
Along with alignment to transformation objectives, establishing governance structures for AI implementation is essential to a sustainable AI strategy. Governance and ethical guidelines ensure accountability and transparency.
Organizations should develop ethical guidelines that dictate how AI technologies are used, addressing concerns related to bias, privacy, and compliance.
Putting these guidelines into action requires a strong AI governance framework. Agentic systems require human supervision to ensure ethical decision-making and regulatory compliance.
- Establish clear protocols for data usage, algorithmic transparency, and bias mitigation.
- Regularly audit datasets to address potential biases.
- Implement mechanisms for human intervention in high-stakes scenarios to ensure decisions align with ethical standards.
Privacy is also critical. Agentic systems often process sensitive information, so following data protection regulations is a must to safeguard organizational integrity and user trust.
Integrating agentic AI into existing operations may also necessitate change management strategies to support employee adoption and effective use. This may involve training programs, communication plans, and ongoing support to help employees adapt to new tools and processes.
Challenges and considerations.
To ensure responsible deployment of agentic AI systems, organizations must address ethical, technical, and regulatory concerns, starting with balancing autonomy and human oversight/controls.
While agentic systems excel at decision-making and automation, unchecked autonomy can lead to unintended consequences. Mitigate risks by implementing governance frameworks defining clear boundaries for AI behavior and include human intervention for decisions that rely on ethical judgment.
Addressing AI bias and fairness
AI bias is a significant concern since agentic systems rely on large datasets that can have underlying biases, which can lead to unfair or discriminatory outcomes.
One of the most effective ways to combat bias in AI systems is to ensure that the datasets used for training are diverse and representative of the populations. Once in operation, use algorithms designed to detect and mitigate bias and refine AI models based on bias detection results.
Along with regularly auditing AI behavior for fairness and accountability, employ human supervision where human subject matter experts assess AI outputs, especially for high-stakes decisions.
And workforce-wide, encourage a culture where team members feel responsible for the ethical implications of AI systems. Providing training on bias and fairness is one way to help empower employees to actively monitor and address these issues.
Privacy and data security
Processing sensitive data raises privacy and cybersecurity concerns. Unauthorized access could expose confidential information, while unsecured APIs might become entry points for attacks.
Address vulnerabilities by adopting advanced encryption, implementing strict access controls, and updating cybersecurity protocols. Comply with data protection regulations to maintain user trust and avoid legal issues.
Navigating regulatory compliance
The regulatory landscape for AI is evolving with new guidelines governing deployment in progress in many jurisdictions. Agentic systems may operate in areas where regulations aren't fully established.
Stay ahead of regulatory changes by participating in industry initiatives and collaborating with policymakers. Proactive compliance minimizes legal risks and positions companies as leaders in responsible AI innovation.
Ethical governance and transparency
Transparency is core to ethical AI deployment. Users need to understand how agentic systems make decisions, especially in applications interacting directly with end users.
Invest in explainable AI techniques to make decision-making more transparent. Clear documentation and stakeholder communication ensure AI initiatives align with organizational values and societal expectations.
The role of automation platforms in agentic systems.
Automation platforms provide the infrastructure and tools to turn AI capabilities into practical applications. They provide the framework to design, deploy, and manage AI agents effectively, at scale, offering a centralized environment where organizations can build, monitor, and optimize agentic systems.
Modern automation platforms come pre-equipped with capabilities that simplify the development and deployment of agentic AI—in particular:
- Low-code development environments. Low-code or no-code development environments empower users to create and customize AI agents without requiring deep technical expertise. This democratization of development allows business users to contribute to AI initiatives and dramatically speeds up the process of building agentic solutions for enterprise-specific applications.
- Built-in connectors and APIs. Integration capabilities are the connective tissue of agentic systems, linking components into cohesive ecosystems. Automation platforms typically include a wide range of built-in connectors and APIs for seamless integration with existing enterprise tools and applications.
- Robust governance and security features. With the increasing focus on responsible AI use and regulatory compliance, modern automation platforms prioritize governance and security. They provide mechanisms for monitoring AI behavior, auditing, refining AI outputs, and maintaining compliance with data protection regulations.
Because integration capabilities are so vital—think of an agentic workflow in supply chain management that links predictive analytics with inventory databases and logistics software—the fact that automation platforms allow AI agents to interact seamlessly and securely across systems and data sources makes them indispensable for any enterprise application of agentic systems.
Building the bridge from RPA to agentic automation
Automation platforms are instrumental in transitioning from traditional, stand-alone, robotic process automation (RPA) to agentic automation. Platforms that combine the reliability of RPA capabilities to execute tasks with the flexibility and autonomy of agentic AI technologies are powering dramatically more intelligent and adaptable workflows.
Integrating RPA and cognitive automation tools with agentic AI allows organizations to automate complex, end-to-end business processes that were previously too unpredictable for traditional automation.
Providing a unified environment for AI technologies
A centralized platform for managing and coordinating multiple AI agents that operate across different business functions is key to maximizing the potential of agentic systems. It’s what enables connecting different AI technologies while maintaining coherence and control.
Automation platforms serve as this unified environment for integrating different AI models and tools to create powerful agentic systems, bridging the gaps between AI capabilities and real-world applications.
Embracing the future with Automation Anywhere.
The transformative potential of agentic AI systems is reshaping the way work gets done. Organizations can now flexibly automate complex workflows with AI agents and accelerate data-driven decisions.
Automation Anywhere is leading this evolution, enabling organizations to harness agentic process automation for unparalleled efficiency, adaptability, responsiveness, and innovation.
With the Agentic Process Automation System, organizations can deploy AI agents with robust automation capabilities and integrate multiple AI models from major cloud providers for optimal agentic workflows.
Making agentic automation accessible to developers of all skill levels, low-code AI Agent Studio democratizes AI agent creation while providing a comprehensive framework to build agents capable of handling complex, multi-step processes involving diverse data sources.
The Automation Anywhere platform emphasizes security, governance, and responsible AI use, providing organizations with the means to implement AI solutions that uphold ethical and regulatory standards. Built-in tools for monitoring, auditing, and refining AI models empower rigorous governance, mitigate risks, and establish trust in AI decision-making.
Ready to unlock agentic AI's full potential and drive meaningful change? Request a demo today.