The autonomous enterprise with APA
The internet and e-commerce were disruptive innovations in the evolution of business, changing business models and value delivery. Similarly, the autonomous enterprise represents a groundbreaking shift, changing how business decisions are made and executed.
The distinction between companies dabbling in automation and those fully embracing autonomy is significant. Many businesses automate 10-30% of processes; an autonomous enterprise operates with over 50% of processes running independently, and up to 80% of work automated overall.
This happens by applying agentic process automation (APA) and AI-driven decision-making to execute core business processes with minimal human intervention.
APA can coordinate and carry out long-running, complex business processes from start to finish across traditionally siloed systems like ERP, CRM, SCM, and finance. It uses smart AI agents that can think, make decisions, and take action on their own to reach goals.
These AI agents work smoothly together, breaking down barriers between data, systems, and departments, and make sense of information as it comes in, like customer feedback, market changes, or operational data, quickly using new information to make decisions and take next steps.
It's important to note that an autonomous enterprise doesn't eliminate human work—it redefines it. Employees transition from managing repetitive tasks to overseeing AI-driven systems, making strategic decisions, and developing new business models, focusing on creativity and strategic growth.
The evolution of enterprise automation
From basic automation to autonomous enterprise, the road from streamlining repetitive tasks to self-driving complex workflows follows a clear progression in step with larger technology developments. Automation tools evolved alongside broader technological developments, including SaaS, cloud, and enterprise AI.
From RPA to APA
Today’s agentic process automation has its roots in robotic process automation (RPA). RPA could handle structured processes with remarkable efficiency. However, its scope was limited. RPA struggled with unstructured data and lacked the cognitive capabilities for decision-making and problem-solving.
The arrival of artificial intelligence (AI) and machine learning allowed automation systems to learn from data, adapt to new situations, and make predictions and informed decisions. This also made possible the automation of processes that rely on unstructured data. In this way, intelligent automation could overcome the limitations of traditional RPA.
Process mining also played a key role, providing visibility into actual process execution versus assumed workflows, identifying automation opportunities by revealing process bottlenecks and enabling continuous process optimization based on real performance data.
However, traditional automation approaches often led to siloed systems, where isolated tasks were automated without considering the broader enterprise context. This created inefficiencies and bottlenecks, limiting scalability and integration.
Agentic process automation sheds these limitations. It breaks down silos, offering a cohesive and integrated approach to automation across all business systems and functions.
To illustrate the journey from RPA to autonomous enterprise, consider a timeline of business process automation technologies:
- Robotic process automation (RPA) represents the initial phase, focusing on automating repetitive, rule-based tasks, usually within specific departments.
- Intelligent automation is the integration of AI and machine learning with RPA to automate more complex processes, including unstructured data and requiring cognitive capabilities.
- Agentic process automation (APA) is the current phase, where over 50% of processes operate autonomously, driven by AI agents and seamless system integration.
Technology advances enable the shift to the autonomous enterprise
Agentic process automation marks a critical inflection point. It brings together multiple technology advancements with automation tools to deliver breakthrough capabilities that power autonomous workflows:
- Natural language processing (NLP): NLP allows automation systems to understand and generate human language, which is key to processing documents, emails, and conversations. NLP also makes it easy for humans to collaborate with automations using natural language.
- Knowledge graphs and semantic technologies: These create structured representations of business knowledge, enabling reasoning across information sources, and supporting contextual understanding of business processes.
- Large language models (LLMs): Bring generalized reasoning capabilities to automations which is what powers the ability to handle novel situations not seen in training data. LLMs also simplify creating automations, allowing development using natural language
- Low-code/no-code platforms: Expands automation development beyond IT specialists and accelerates deployment of automation solutions. Importantly, it enables business users to participate in designing automations.
- API ecosystems and microservices: The unsung heroes of enterprise automation, APIs provide standardized connections between systems, enable modular automation design that can be reconfigured, and support integration across previously siloed systems
- Event-driven architectures: This means that automations can respond to real-time business events. It also enables coordination across multiple AI agents and supports asynchronous process execution.
- Agentic AI: AI agents and agentic systems build on all of these technologies to bring autonomy, adaptability, and goal orientation to automations so that they can pursue objectives with more independence, making decisions about how to achieve goals rather than just following fixed procedures. This enables handling complex, variable processes that would be impossible to fully specify in advance.
These technologies all contribute to the evolution of automation from mimicking human interface actions to understanding business context, making decisions, taking action, and adapting to new situations—the hallmarks of true agentic process automation—which powers the autonomous enterprise.
Tour the secure Agentic Process Automation System
Core components of an autonomous enterprise
At the heart of an autonomous enterprise lies the concept of agentic process automation (APA). APA is a groundbreaking approach to business process automation that employs AI agents to manage end-to-end processes with minimal human intervention.
These AI agents are designed to mimic human decision-making, allowing them to navigate complex workflows and execute tasks autonomously. Combining AI capabilities with action, these systems can process unstructured data, recognize patterns, and make data-driven decisions.
Unlike traditional automation solutions that tend to focus on vertical point solutions—meaning, they address isolated tasks within specific departments—APA delivers horizontal integration. AI agents work seamlessly across applications, creating a cohesive and interconnected enterprise ecosystem.
This horizontal approach eradicates the invisible barriers between different parts of the organization, reorienting them towards common strategic goals, efficiency, and productivity.
Orchestration plays a central role here, managing multiple automation systems and AI agents within the autonomous enterprise. It involves coordinating and synchronizing different processes and systems so they cooperate seamlessly. Effective orchestration means that AI agents can communicate and collaborate across different platforms, preventing conflicts and redundancies and optimizing resource use.
Collaboration between human workers and autonomous systems
In an autonomous enterprise, human workers collaborate with autonomous systems by providing the strategic direction and context that AI agents need to function effectively. This teamwork between human workers and agentic automation is key to unlocking efficiency and innovation.
While AI agents are great at handling tasks and making decisions based on data, the human element remains essential for oversight and creative problem-solving. Humans set the overarching goals and parameters and make sure automated processes align with the organization's values and objectives.
With AI agents taking care of repetitive and data-heavy tasks, human workers can focus on areas that need emotional intelligence, creativity, and strategic thinking. This collaboration is not about replacing people; it's about leaping far beyond current constraints toward greater innovation and competitiveness.
“The autonomous enterprise enables employees to focus on what matters most—delivering business value.”
—Forbes
Moreover, human workers are the source of truth for monitoring and refining the performance of AI agents. By analyzing the outcomes and providing feedback, they can continuously improve the systems, ensuring they adapt to changing business needs and environments.
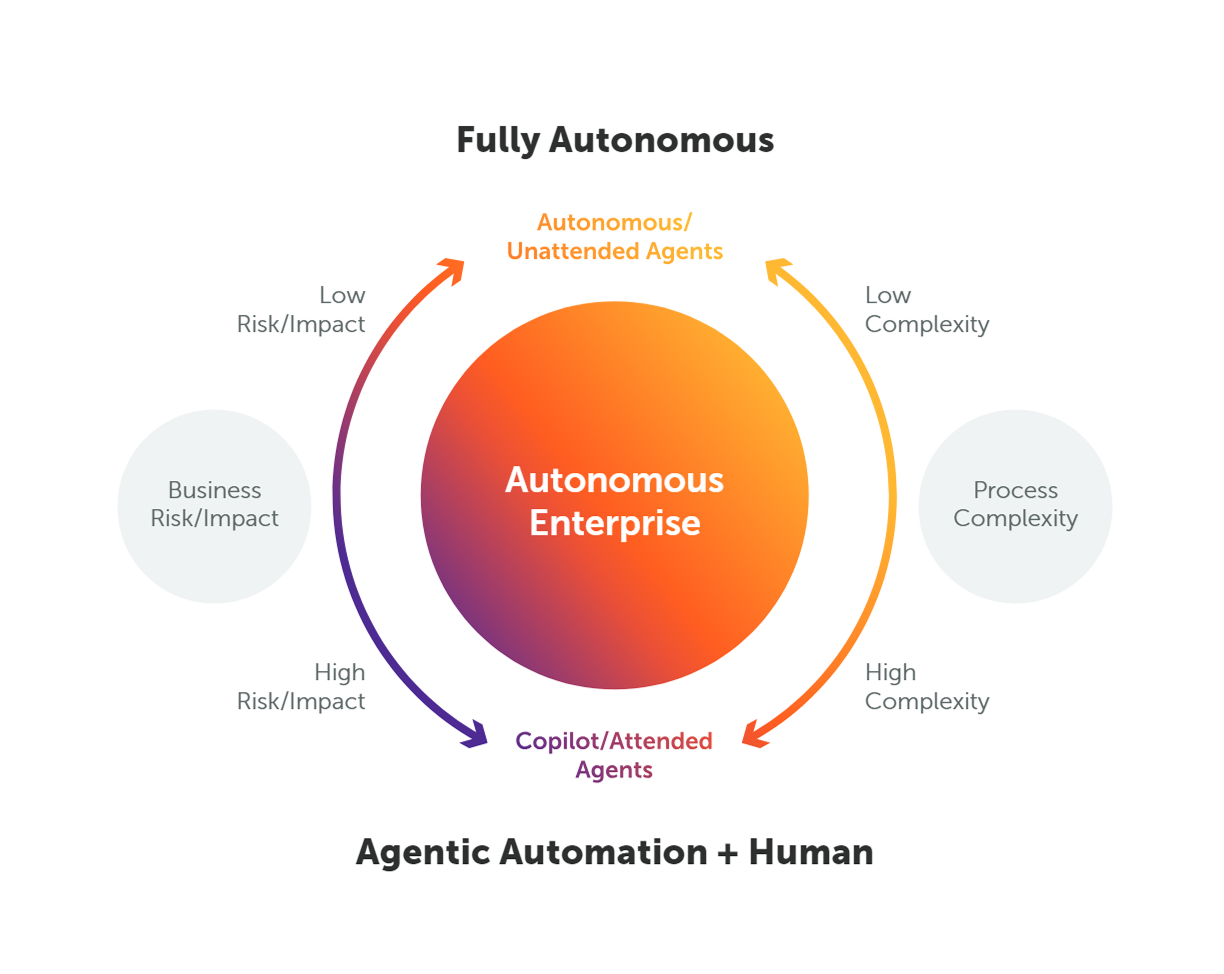
Business benefits of becoming an autonomous enterprise
Becoming an autonomous enterprise fundamentally reshapes how organizations use resources and drive growth. Embracing autonomous workflows delivers multifaceted business benefits, impacting budget allocation, workforce dynamics, operational accuracy, and customer satisfaction.
Shifting budget from maintenance to innovation
For Chief Information Officers (CIOs), this transformation is particularly significant as it allows a strategic shift in budget allocation from routine maintenance to innovation initiatives. Traditionally, a large portion of IT budgets is consumed by "keeping the lights on"—maintaining existing systems and infrastructure.
With agentic process automation, enterprises can have self-managing IT systems. This shift allows CIOs to invest in innovative projects that drive business growth, such as developing new products, entering new markets, or enhancing customer experiences. As a result, organizations can scale and pivot more rapidly, adapting to market changes and seizing new opportunities for sustained growth.
Elevating employee roles to drive revenue growth
One of the most profound impacts of APA is its ability to elevate the workforce from repetitive, manual tasks to high-value roles. This transition doesn't just reduce operational costs; it actively drives revenue growth. Employees shift to strategic activities that require human creativity, problem-solving, and decision-making. Tackling innovation and customer engagement, the workforce is able to use their skills to create new business opportunities and enhance competitive advantage.
Enhancing accuracy, compliance, and risk management
Autonomous enterprises benefit from significant improvements in accuracy, compliance, and risk management. AI systems excel at processing large volumes of data, reducing the chance of errors that can occur with manual handling. This accuracy is a boost for maintaining compliance with industry regulations and standards. Plus, AI agents can be programmed to adhere to regulatory requirements and corporate policies without fail.
And AI's ability to anticipate potential issues and implement preventative measures significantly bolsters risk management. AI-driven analytics provide real-time insights into potential risks, enabling proactive management and mitigation. With continuous monitoring and data analysis, autonomous systems can identify anomalies and take corrective actions, ensuring the enterprise operates within legal and ethical boundaries.
Improving customer experience
The benefits of becoming an autonomous enterprise extend to the customer experience as well. In fact, improving customer experience is often the driving force behind initial agentic process automation projects.
The autonomous enterprise delivers faster and more consistent service to customers. AI systems can process customer requests in real time, providing immediate responses and solutions. This leads to shorter wait times and higher customer satisfaction.
Automated systems make sure that service delivery is consistent across all touchpoints, maintaining the same high standards regardless of time or channel. This reliability builds customer trust and loyalty because customers know they can expect the same prompt and accurate service every time they interact with the enterprise.
Real-world examples and use cases
In the journey towards becoming an autonomous enterprise, businesses are leveraging AI-driven automation to revolutionize traditional workflows and enhance operational efficiency across domains. This transformative shift is exemplified by the integration of AI agents in critical operations processes, but also in areas like sales and marketing, where less structured, traditionally human-driven processes use agentic process automation to achieve better, faster outcomes.
This shift is further illustrated by real-world case studies, where organizations like Petrobras, Boston Children’s Hospital, and KeyBank have harnessed agentic process automation to achieve remarkable improvements in efficiency, accuracy, and customer satisfaction.
Case studies
Petrobras: Revolutionizing tax management
Petrobras, a leading energy company in Brazil, harnessed the power of agentic process automation to tackle the complex and ever-changing landscape of tax management. Each year, Petrobras faces the daunting task of deciphering thousands of tax code changes, a process that traditionally required significant manual effort and time.
By implementing APA, Petrobras automated the analysis and application of these tax changes, allowing AI agents to rapidly recompute taxes with unparalleled accuracy. This automation not only streamlined the tax management process but also resulted in substantial financial savings: In just three weeks, Petrobras saved over $100 million.
“We filed our taxes in three days. For the first time in 15 years, we didn’t have to work over the weekend to file taxes.”
—Victor Pace, General Manager of Taxes & Government Participations, Petrobras
Boston Children’s Hospital: Enhancing physician efficiency
Boston Children’s Hospital leveraged agentic process automation to address the administrative burdens that often weigh heavily on healthcare professionals. Complex pre- and post-visit administrative tasks, such as scheduling, billing, and documentation, can consume up to 25% of a physician’s time, detracting from patient care.
By deploying APA, the hospital automated these time-consuming processes, significantly reducing the administrative workload for physicians. This automation not only improved operational efficiency but also enhanced physician well-being by allowing them to devote more time and attention to the patient experience. As a result, Boston Children’s Hospital has seen improvements in both staff satisfaction and patient outcomes, illustrating the positive impact of APA in the healthcare sector.
KeyBank: Streamlining financial operations
KeyBank, a major financial institution, utilized agentic process automation to transform its operational efficiency across the organization. Faced with the challenge of managing numerous repetitive and time-intensive processes, KeyBank implemented APA to automate nearly 300 processes across departments.
These automated processes span tasks such as transaction processing, compliance checks, and customer service inquiries. By automating these workflows, KeyBank not only improved business efficiency but also reduced the risk of human error and ensured compliance with regulatory standards. The implementation of APA has allowed KeyBank to operate more smoothly and responsively, providing better service to its customers and positioning itself as a leader in financial innovation.
Sales and Marketing
While sales and marketing may not immediately be recognized as prime candidates for autonomous processes, the value and impact of agentic process automation are high for these departments.
For example, AI agent-driven automation can streamline the quote generation process. Typically, generating quotes involves gathering data from multiple sources, calculating costs, checking accuracy, and seeking approvals—which is prone to delays.
With autonomous systems, AI agents can instantly pull relevant data, such as product specifications, pricing, and customer information, from all databases. They apply rules and algorithms to calculate accurate quotes in real time, ensuring compliance with pricing strategies and discount policies.
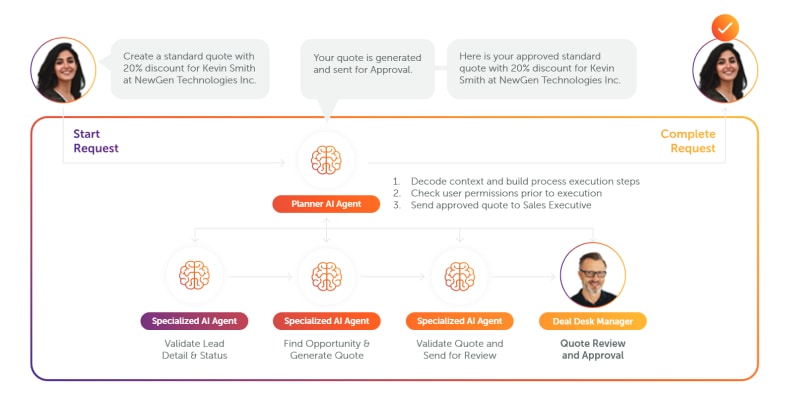
This agentic automation accelerates quote generation while boosting accuracy and consistency, and reducing the risk of costly errors. And AI-driven insights can provide sales teams with recommendations for optimal pricing and discount opportunities, further enhancing their ability to meet customer needs and drive revenue growth.
And in marketing, AI agents can accelerate the content creation and publishing process. Developing and distributing content usually involves substantial manual effort, from brainstorming ideas and drafting copy to scheduling and publishing across platforms.
AI agents streamline this workflow by generating high-quality content that resonates with target audiences. By analyzing data—consumer preferences, search trends, and competitor activity—AI systems can suggest relevant topics and create compelling content tailored to specific audience segments.
And AI systems can automate the scheduling and publishing process, optimizing timing for maximum engagement across multiple channels, such as social media, blogs, and email campaigns. This automation not only accelerates the content pipeline but also enhances precision and reach.
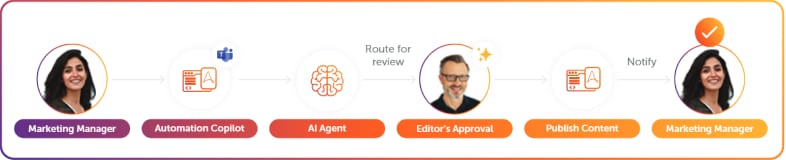
The human element in an autonomous enterprise
The idea of an autonomous enterprise can conjure an image of mass unemployment, a misconception that warrants direct attention. This transformation is not a zero-sum game; it's an evolution that benefits both the organization and its workforce.
The human element in an autonomous enterprise is about collaboration and growth. While AI and automation handle tasks, they simultaneously open avenues for employees to focus on high-value activities.
Augmentation vs. replacement
The concept of augmentation versus replacement is central to understanding what an autonomous enterprise means for humans.
At its core, augmentation refers to the enhancement of human capabilities through technology, allowing individuals to perform tasks more efficiently and effectively. This contrasts with replacement, where technology completely takes over tasks traditionally performed by humans, often leading to concerns about job displacement.
In an autonomous enterprise, augmentation is about leveraging technology to supercharge human skills, rather than rendering them obsolete. For instance, AI-driven tools can handle repetitive and data-intensive workflows, allowing employees to focus on strategic, creative, and interpersonal activities that require human intuition and emotional intelligence. The idea of this relationship between humans and technology is about realizing productivity and innovation gains, enabling employees to devote more time to creative problem-solving and decision-making.
Augmentation is also about continuous learning and adaptation. As technology evolves, so do the skills required to work alongside it. Organizations that prioritize augmentation—and the training and upskilling to support it—are more likely to cultivate a motivated and engaged workforce, as employees will remain valued and integral to the enterprise's success.
Many organizations have already implemented reskilling and upskilling programs to support this transition. These programs are core to preparing employees for new roles being created by autonomous systems.
As a case in point, SoftBank has a strategic focus on upskilling its workforce so that employees are equipped to maximize AI's potential. Training programs aim to expand employee skills in areas like AI integration, data analysis, and process automation, empowering employees to transition from routine tasks to more value-added roles. The excitement from the workforce is palpable; an idea contest for utilizing generative AI to enhance employee experience generated 52,000 proposals in ten days!
“We aimed to inspire a fresh sensibility through the experience of technology... we have been realizing a work style and workplace where employees and technology are integrated together.”
—Satoshi Kaminagayoshi, VP of Customer Success, SoftBank Corp.
Importantly, the augmentation approach also aligns with ethical considerations and societal expectations. By focusing on extending what’s possible with human capabilities, rather than replacing them, autonomous enterprises can mitigate potential job losses and contribute to economic stability. This also relates to concerns about the dehumanization of work; augmentation places value on human creativity and judgment in driving business outcomes.
“ We already see a shift in employee preferences. Job seekers now consider AI adoption when choosing employers. No one wants to work for a company that relies on outdated manual processes, as it doesn’t contribute to career growth. Employees want careers, not just jobs. Employers will have to recognize this too and use AI as a competitive advantage that enhances their operations.”
—Mihir Shukla, Founder and CEO, Automation Anywhere
Common challenges and how to overcome them
As enterprises transition towards autonomy, they will encounter challenges. Among these, addressing skills gaps and the governance and oversight of autonomous systems stand out as critical considerations. Ensuring that AI-driven processes align with organizational values and regulatory requirements is paramount.
Beyond these, companies may face hurdles such as integrating complex AI systems with existing infrastructure, which often requires significant technological upgrades and investments. Data management is another critical challenge, as enterprises must ensure robust data quality, security, and privacy to support autonomous operations. Additionally, aligning AI-driven processes with organizational goals and values is essential to maintain coherence and drive strategic success.
Governance and oversight considerations for autonomous systems
Establishing robust governance frameworks is essential to maintain control over automated operations, ensuring transparency, accountability, and ethical compliance. Companies should implement oversight mechanisms that include regular audits, compliance checks, and ethical reviews to safeguard against potential risks.
Regulatory frameworks
- Develop comprehensive guidelines addressing data privacy, security, and ethical use.
- Ensure compliance with legal standards to protect user rights.
- Establish policies for data collection, storage, and usage, with mechanisms to prevent unauthorized access and mitigate cyber threats.
Accountability
- Designate who is responsible for the performance and outcomes of autonomous systems.
- Implement regular audits and evaluations to ensure adherence to guidelines and identify improvement areas.
Transparency and explainability
- Provide clear insights into the decision-making processes of autonomous systems.
- Explain how systems operate, the data used, and the rationale behind decisions to build stakeholder trust.
Human oversight and intervention
- Incorporate mechanisms for human intervention in cases of system failure or ethical dilemmas.
- Ensure human judgment remains integral to decision-making, especially in complex, high-stakes, or unforeseen scenarios.
Overcoming skills gaps
Another significant challenge is addressing skills gaps within the workforce. As businesses adopt advanced technologies, the demand for new skills increases and accelerates, which means organizations need to proactively develop talent to thrive in an autonomous enterprise.
This involves investing in comprehensive training and development programs that focus on digital literacy, AI management, and advanced analytics. Collaborations with educational institutions and online learning platforms can also help facilitate continuous learning and skills development so that employees are equipped for the demands of an evolving workplace.
Assessment and strategic planning
- Conduct regular, data-driven skills assessments to map current capabilities against future requirements
- Identify priority areas where training will deliver the most significant business impact
- Develop skills taxonomies that provide clear visibility into organizational capabilities and gaps
Personalized development pathways
- Create customized learning journeys that align individual career aspirations with organizational needs
- Implement AI-powered learning platforms that adapt content based on individual progress and learning styles
- Balance technical skills (data science, programming) with human capabilities (critical thinking, ethical judgment)
Incentives and culture
- Establish meaningful reward systems tied to skills acquisition and application
- Allocate dedicated time for learning within standard work schedules
- Foster a culture where continuous improvement is part of everyday operations
Implementation and partnership strategies
- Build internal skills marketplaces that allow employees to apply new capabilities on cross-functional projects
- Form strategic alliances with educational institutions and specialized training providers
- Create hybrid teams that pair domain experts with technical specialists to accelerate knowledge transfer
Measuring progress and impact
- Track skills development through metrics directly connected to business outcomes
- Monitor how effectively new capabilities translate into improved operational performance
- Assess the ROI of training initiatives through productivity gains and innovation metrics
Ultimately, a central takeaway is to recognize that a successful transition to an autonomous enterprise requires both technical competency and the uniquely human skills that complement automation.
While the journey to becoming an autonomous enterprise presents challenges, prioritizing governance and investing in talent development will position organizations to succeed.
The problem with siloed AI deployment
Deploying AI in vertical silos, such as customer relationship management (CRM) or enterprise resource planning (ERP) systems, significantly limits the potential for transformation.
When AI is confined to specific functional areas, it exacerbates existing barriers to productivity; siloed deployment often results in fragmented insights and suboptimal decision-making, as data and processes remain disconnected, isolated within individual departments. This narrow focus restricts the ability to create cohesive, enterprise-wide strategies.
To illustrate, consider the impact of implementing AI within a CRM system for a sales team. Suppose a global company with 70,000 employees, 15% of whom are in sales, integrates AI to enhance CRM efficiency. Even with aggressive assumptions—such as AI augmenting a full 50% of CRM-related tasks and three-quarters of sellers adopting it consistently for a 25% productivity boost—the result is only a 1.7% increase in sales team efficiency.
What’s more, this translates to a mere 0.3% improvement in overall company efficiency.
This minimal gain highlights the limited impact of AI when applied within a single system or function. It fails to address the broader operational challenges and opportunities that span the entire enterprise.
Unfortunately, vendor-specific AI solutions often perpetuate these silos rather than dismantling them. When organizations rely on AI tools tailored to particular platforms or ecosystems, they inadvertently reinforce the separation between different functional areas. This approach limits the ability to integrate AI and autonomous processes across departments.
To achieve an autonomous enterprise, organizations must apply an integrated approach that deploys AI across all facets of the business. Instead of perpetuating silos, organizations should seek out AI solutions that promote horizontal integration and seamless communication between different business units.
Steps to evolve into an autonomous enterprise
Embarking on the journey to becoming an autonomous enterprise can be described as a structured progression through key stages, each marked by distinct roles for humans and AI. This evolution requires a strategic approach that integrates technology, organizational change, and human-machine collaboration.
Stages of the autonomous enterprise evolution
Stage 1: Initial human-led AI assistance
At this foundational stage, organizations begin by leveraging basic AI tools to support human decision-making. Characterized by significant human oversight, AI is used in data analysis, enhanced software, and decision support systems. For example, customer support chatbots handle simple inquiries, freeing human agents for more complex issues. This stage is about learning, experimentation, and exploration.
Stage 2: Emerging collaboration from AI-augmented decisions
Here, AI enhances human decision-making by providing valuable insights. Organizations adopt advanced analytics platforms and machine learning tools to augment processes. For instance, financial institutions use AI to analyze market trends and suggest investment opportunities, allowing analysts to make informed decisions. This stage focuses on AI-powered productivity tools and intermediate AI collaboration techniques.
Stage 3: Balanced collaboration between humans and AI
At this stage, humans and AI collaborate seamlessly, sharing decision-making responsibilities. Organizations deploy integrated AI systems, predictive analytics, and collaborative tools. In healthcare, AI assists physicians by analyzing patient data to recommend personalized treatment plans, with physicians providing final decisions. This stage emphasizes collaborative problem-solving with AI.
Stage 4: Advanced collaboration, AI-led with human oversight
AI takes the lead in providing information and executing processes, relying on human supervision for critical decisions. In manufacturing, AI autonomously manages production lines while human oversight ensures quality control. This stage involves autonomous AI systems managing complex operations and requires advanced AI strategy development.
Stage 5: Autonomous enterprise with fully autonomous operations
At the most mature stage, operations function independently with self-learning AI platforms and autonomous decision-making systems managing processes with minimal human intervention. Humans focus on strategic tasks and AI governance. For example, a retailer uses AI to manage store operations, freeing humans for in-store processes. This stage requires expertise in AI governance and understanding autonomous decision-making.
Roles of humans and AI at each stage
Stage | Description | Role of Humans | Role of AI and Agentic Process Automation | |
1 | Stage 1: Human-led with AI assistance | Basic AI tools support human decision-making. Significant human direction and oversight. | Lead decision-making with AI for data analysis and support. Explore AI capabilities through learning and experimentation. | AI tools assist in data analysis and decision support, handling simple tasks. |
2 | Stage 2: Collaboration and AI-augmented decisions | AI enhances human decision-making. Use of advanced analytics platforms and ML tools. | Make informed decisions based on AI insights, focusing on strategic analysis and decision-making. | AI augments processes by analyzing data and suggesting opportunities. |
3 | Stage 3: Human-AI teamwork | Humans share decision-making with integrated AI systems and predictive analytics. | Work collaboratively with AI, providing final decisions and oversight. | AI assists in decision-making, analyzing data and recommending actions. |
4 | Stage 4: Advanced collaboration, AI-led with human oversight | AI leads process execution. Human supervision for critical decisions. | Oversee AI-led operations, ensuring quality and managing exceptions. | AI autonomously manages complex operations, requiring minimal human intervention. |
5 | Stage 5: Autonomous enterprise | Operations run independently with self-learning AI platforms. Minimal human intervention. | Focus on strategic tasks and AI governance, overseeing autonomous systems. | AI autonomously manages processes and decision-making, continuously learning and adapting. |
Essential technology capabilities at each stage
At each stage, organizations must develop the technology capabilities to support the progression to more autonomous operations. Initially, basic automation tools are sufficient to handle repetitive tasks. As organizations progress, intelligent systems with AI agents are needed to enable decision-making and process orchestration across the business.
In the first stage, where AI is introduced to assist human-led tasks, technology needs are primarily for automation, data management, and analysis to support decision-making.
- Basic automation tools: Foundational automation tools like RPA to handle repetitive tasks and streamline unambiguous processes
- Data collection and management systems: Systems for collecting, storing, and managing data efficiently, ensuring data quality and accessibility.
- Decision support systems: Systems that assist humans with making decisions by providing relevant data and insights.
By stage 2, where collaborative human-AI workflows are starting to emerge, along with AI-augmented decisions, technology requirements include more advanced integration and analytics capabilities.
- Advanced analytics tools: Platforms capable of complex data analyses to provide deeper insights and support decision-making.
- Machine learning models: Models to identify patterns and trends and provide predictive insights to augment human decisions.
- Integration capabilities: Integration capabilities to connect disparate systems and data sources for seamless information flow.
At stage 3, technologies must support a shared operating environment where collaboration between humans and AI begins to feel frictionless.
- Predictive analytics and AI systems: Tools that enable proactive decision-making and risk management.
- Collaborative tools: Platforms that support seamless collaboration between humans and AI, enhancing teamwork and decision-sharing.
- Data visualization: Tools that present data in intuitive, easily understandable formats to support collaborative analysis.
To move toward more advanced collaboration and AI-led execution with human oversight—as in stage 4—agentic process automation is designed to provide the necessary AI flexibility, integration, and orchestration.
- Agentic AI systems:AI systems capable of autonomous decision-making and process execution, with built-in mechanisms for human oversight.
- Real-time data processing: Capabilities for real-time data processing and analysis, enabling immediate insights and responses.
From here, the bridge to fully autonomous operations starts with AI strategy development, aligning organizational goals and AI-led operations. This strategy must be developed in tandem with AI governance frameworks to establish robust AI governance that ensures ethical, compliant, and transparent AI operations.
- Self-learning AI: Platforms that continuously learn and adapt to changing environments, optimizing operations autonomously.
- Integrated AI agents: Agentic AI agents that manage complex operations and make strategic decisions with minimal human intervention.
Organizational evolution
Organizational structure must evolve in parallel with the adoption of these technologies.
This evolution begins at the foundational stage, where departments often operate in silos, mostly focusing on specific tasks with limited cross-departmental collaboration. At this starting point, the organization is exploring AI capabilities, with humans performing the majority of tasks and AI tools providing support in data analysis and decision-making.
With increasing AI-driven data sharing and cross-functional insights, collaboration across departments becomes more frequent, which begins to break down traditional silos, setting the stage for a more integrated approach. Cross-functional teams may emerge, working collaboratively with AI systems to achieve shared goals, with a shift to humans and AI sharing decision-making responsibilities.
As the organization evolves, it embraces cross-functional ownership of processes, with integrated teams responsible for overseeing AI-led operations. This approach ensures alignment with strategic objectives and encourages a culture of continuous improvement.
As processes become more autonomous, humans transition to roles focused on strategic tasks, innovation, and AI governance, overseeing AI-led processes to ensure quality and strategic alignment. Throughout this journey, the human-machine relationship transforms significantly. Initially, humans perform the bulk of the work, with AI handling simple tasks. As systems become more sophisticated, humans transition to monitoring and overseeing autonomous processes, focusing on strategic governance and policy oversight.
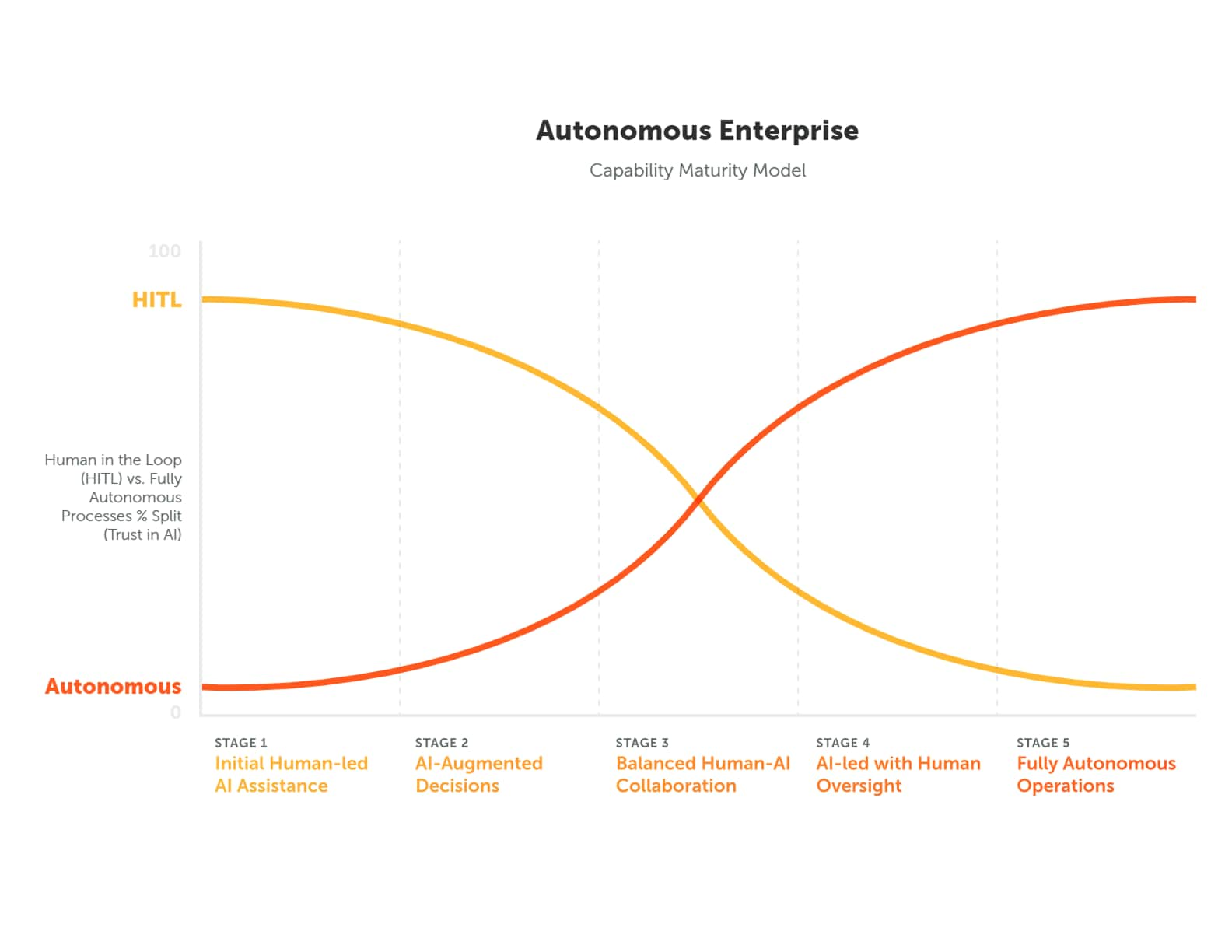
Ultimately, the organization evolves into an autonomous enterprise characterized by integrated process ownership and seamless partnership between humans and AI.
Initially, departments may operate in silos, but as AI systems integrate across the enterprise, cross-functional process ownership becomes essential. This shift fosters collaboration and ensures that AI solutions are aligned with broader business objectives.
Where to start and achieve quick wins
Kickstart the transformation by focusing on areas that can yield early wins. It’s important to gain buy-in and help all stakeholders see the value, along with providing realistic timeframes for achieving results and making major evolutionary leaps.
Areas where organizations might find early wins:
Basic process automation: Automate simple, repetitive processes that are time-consuming for employees. This could include automating data entry, invoice processing, or routine customer inquiries. These tasks often yield quick efficiency gains and cost savings.
Data-driven insights: Leverage AI to analyze existing data for valuable insights to inform decision-making. For example, using AI analytics to identify trends in customer behavior or operational performance can lead to more informed strategies and immediate improvements.
Enhanced decision support: Implement AI tools that assist in decision-making with predictive analytics and recommendations. This can be particularly beneficial in areas like supply chain management or financial forecasting, where accurate predictions can lead to significant improvements in efficiency and cost management.
Improved customer interactions:Deploy agentic AI-powered chatbots or assistants to handle common customer service processes. This not only enhances customer satisfaction by providing quick responses but also allows human agents to focus on more complex issues.
Resource optimization: Use AI to optimize resource allocation, such as workforce scheduling or inventory management, to reduce waste and improve overall productivity.
Key challenges and how to overcome them at each stage
Stage | Key Challenges | Practical Strategies to Overcome Challenges | |
1 | Stage 1: Human-led with AI assistance | - Employee concerns about job security | - Provide training and education to build AI literacy and demonstrate the benefits of AI. - Focus on augmentation, not replacement—train employees for APA-powered roles. |
2 | - Limited understanding of AI capabilities | - Conduct workshops and pilot projects to showcase AI's potential and build confidence. | |
3 | Stage 2: Collaboration and AI-augmented decisions | - Data quality and accessibility issues | - Implement data governance policies to ensure high-quality, accessible data. - Use APA to process unstructured and real-time data—eliminating the need for perfect data. |
4 | - Integration with existing systems | - Use middleware solutions to facilitate integration and ensure seamless data flow. | |
5 | Stage 3: Human-AI teamwork | - Ensuring effective collaboration between humans and AI | - Foster a culture of collaboration and provide tools that support teamwork and communication. |
6 | - Aligning AI initiatives with business goals | - Establish clear objectives and KPIs to measure the impact of AI initiatives on business outcomes. | |
7 | - AI adoption in silos | - Deploy APA as an enterprise-wide orchestration layer, not just AI in individual apps. | |
8 | Stage 4: Advanced collaboration, AI-led with human oversight | - Managing AI governance and ethical considerations | - Develop comprehensive AI governance frameworks and ethical guidelines to ensure responsible AI use. - Implement AI-driven compliance and automated auditability with APA. |
9 | - Maintaining human oversight of AI processes | - Implement monitoring systems and feedback loops to ensure AI processes align with organizational values. | |
10 | Stage 5: Autonomous enterprise | - Ensuring AI systems remain aligned with strategic objectives | - Regularly review and update AI strategies to align with changing business goals and market conditions. |
11 | - Addressing potential biases in AI algorithms | - Conduct regular audits of AI systems to identify and mitigate biases, ensuring fairness and equity. |
Key metrics for measuring progress
Measuring progress toward becoming an autonomous enterprise should extend beyond basic efficiency metrics; it requires a comprehensive set of metrics that capture the multifaceted nature of this transformation. Consider tracking improvements in decision-making speed, process integration, and employee engagement to gauge the broader impact of autonomy.
Organizational readiness
- AI adoption rate: Measure the percentage of processes and operations that have integrated AI technologies. Higher adoption rates indicate progress toward autonomy.
- Skill development and training: Track the number of employees trained in AI-related skills and the completion rates of AI training programs. This reflects the organization's readiness to embrace AI.
- Change management success: Evaluate the effectiveness of change management initiatives through employee feedback and engagement levels in AI projects.
Technology sophistication
- AI system integration: Assess the extent to which AI systems are integrated across different departments and functions. Full integration signifies a move toward seamless operations.
- Data use and quality: Monitor the quality and utilization of data in AI processes. High-quality data that is effectively used in decision-making is crucial for autonomy.
- Innovation rate: Measure the frequency and impact of new AI-driven innovations introduced within the organization.
Operational capability
- Process automation level: Determine the percentage of processes that are fully or partially automated by AI. This metric helps gauge the level of operational autonomy.
- Decision-making speed and accuracy: Evaluate how AI impacts the speed and accuracy of decision-making processes. Faster and more accurate decisions indicate effective AI integration.
- Error reduction rate: Monitor the decrease in errors or defects in operations due to AI intervention, showcasing improved operational capabilities.
Collaborative intelligence
- Human-AI collaboration effectiveness:Measure the efficiency and effectiveness of collaborative efforts between humans and AI. Surveys and performance assessments can provide insights into this dynamic.
- AI-driven insights utilization: Track how often AI-generated insights are used in strategic decision-making. Frequent utilization indicates reliance on AI for critical decisions.
Strategic alignment
- Alignment with business goals: Assess how well AI initiatives align with the organization's strategic objectives. This ensures that AI efforts contribute to broader business success.
- Customer satisfaction and experience: Measure changes in customer satisfaction and experience due to AI-driven improvements. Enhanced customer outcomes reflect successful AI deployment.
Autonomous evolution vs. traditional automation
The journey toward an autonomous enterprise is marked by significant differences in both approach and outcomes when compared to traditional automation implementations.
Approach
In terms of approach, autonomous evolution emphasizes holistic integration of AI across the entire organization. This involves creating interconnected systems that enhance overall operational intelligence and embedding AI into strategic decision-making processes. It includes a focus on continuous improvement and innovation.
In contrast, traditional automation typically targets specific tasks or processes in isolation, aiming to improve efficiency in discrete areas without necessarily considering the broader organizational context or strategic alignment.
Another key difference lies in the relationship between humans and technology. Autonomous evolution centers on collaborative intelligence, where AI augments human capabilities. The goal is orders-of-magnitude improvements in speed, decision-making, creativity, and problem-solving through AI support, through a synergistic partnership between humans and machines.
Traditional automation, on the other hand, often aims to replace human labor in repetitive tasks, focusing on cost reduction and efficiency gains. It typically lacks emphasis on enhancing human roles.
Additionally, the autonomous evolution is about enabling organizations to respond proactively and with agility and speed to new circumstances. This involves AI systems that continuously learn and adapt to changing conditions. Whereas, traditional automation relies on predefined rules and processes that remain static unless manually updated, limiting the ability to adapt quickly to changes and potentially resulting in outdated or inefficient operations.
Outcomes
The outcomes of these approaches also diverge. Autonomous evolution drives strategic transformation by aligning AI initiatives with business goals. This alignment leads to significant improvements in innovation, customer experience, and competitive advantage, focusing on long-term value creation and sustainable growth.
In contrast, traditional automation primarily delivers incremental efficiency gains, such as cost savings and productivity improvements, without necessarily contributing to broader strategic objectives or transformative change.
Autonomous evolution also enhances decision-making capabilities by providing real-time insights and predictive analytics. This empowers organizations to make informed, data-driven decisions that drive success. Traditional automation, on the other hand, streamlines operations by automating routine tasks but may not significantly impact decision-making processes or strategic planning.
And importantly, autonomous evolution encourages a cultural shift toward innovation, collaboration, and continuous learning. This fosters an environment where AI is seen as a partner in achieving organizational goals. Traditional automation focuses on optimizing existing processes, often without addressing the cultural or organizational changes needed to fully leverage AI's potential.
The journey starts with Automation Anywhere
The autonomous enterprise is not just about leveraging AI; it’s about reimagining the very fabric of operations and setting a new standard for what organizations can achieve.
Today, the largest productivity opportunities are long-running, horizontally complex processes that touch many systems and teams, but most AI solutions deploy in vertical silos. AI that works only within specific app ecosystems, like CRM or ERP, adds limited value—and further isolates functions and processes.
Agentic process automation (APA) does the opposite. It connects all systems and coordinates AI agents across operations, creating a productivity snowball effect.
Automation Anywhere is a pioneer in APA, the first to deliver an Agentic Process Automation System, automating exactly these complex, mission-critical, processes at scale.
Our solutions provide the infrastructure for the journey to autonomy. And already deliver breakthrough results for customers who are seamlessly automating complex, long-running processes across departments, vendors, and applications—weaving AI into every aspect of enterprise operations.
Start your journey to an autonomous enterprise. Connect with us and see how agentic process automation works in real time with a personalized live demo.